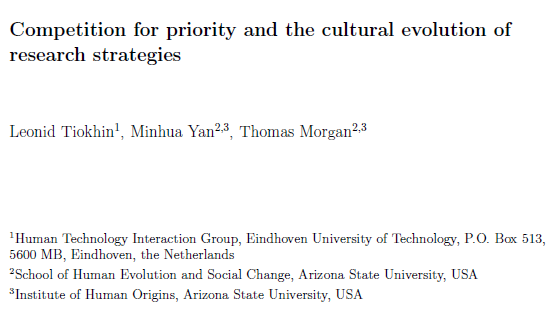
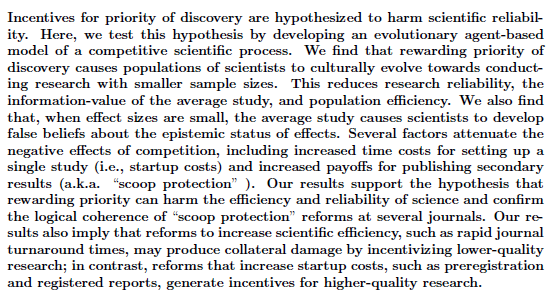
cos.io/prereg/
cos.io/rr/
incentivize scientists to conduct higher-quality research than they would otherwise*
nature.com/articles/31225
royalsocietypublishing.org/doi/abs/10.109…
@wfrankenhuis1 @EikoFried @IrisVanRooij @zerdeve @STWorg @rlmcelreath @CT_Bergstrom @jevinwest @KevinZollman @RemcoHeesen @mmuthukrishna @siminevazire. I'm sure there are many others who I'm blanking on - sorry in advance.