Tweet summary of my new paper with @jenniferdoleac
"Algorithmic Risk Assessment in the Hands of Humans"
papers.ssrn.com/sol3/papers.cf…
1/
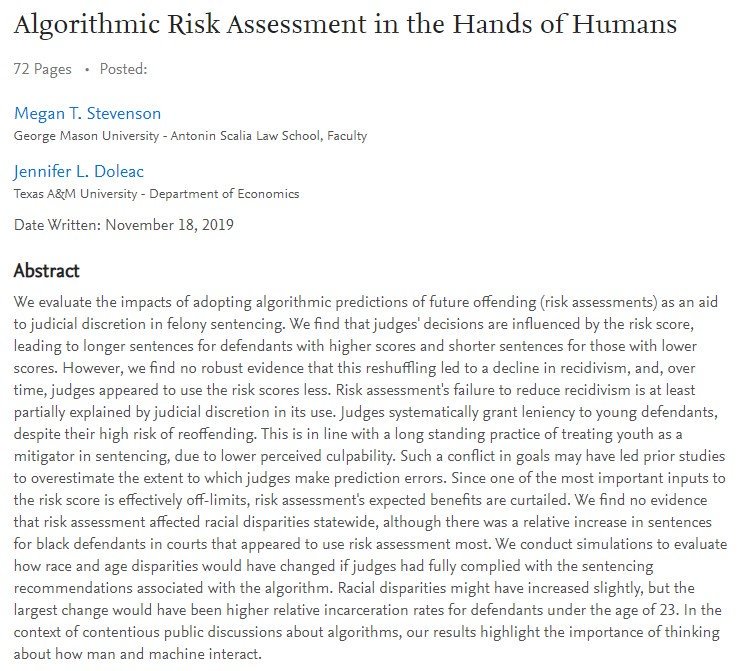
academic.oup.com/qje/article-ab…
papers.ssrn.com/sol3/papers.cf…
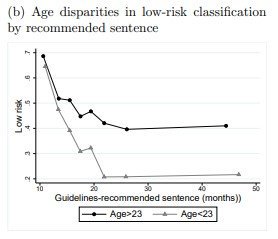
Keep Current with Megan Stevenson
This Thread may be Removed Anytime!
Twitter may remove this content at anytime, convert it as a PDF, save and print for later use!
1) Follow Thread Reader App on Twitter so you can easily mention us!
2) Go to a Twitter thread (series of Tweets by the same owner) and mention us with a keyword "unroll"
@threadreaderapp unroll
You can practice here first or read more on our help page!