My prediction was spot on. Current tally is 20,600.
1/
I became interested in, and very concerned about, the spread of #coronavirus since January 2020, when I saw the initial datasets from #Wuhan.
2/
3/
Ex: one of my papers below, in Science, on modeling climate feedbacks:
doi.org/10.1126/scienc…
4/
Dynamical System: A system of interconnected variables that evolves over time. Ex: pendulum; electric circuit; infectious disease; weather; the Solar System.
5/
6/
7/
8/
9/
10/
#Taiwan managed to CONTAIN the virus and keep the total infections very low (385).
#Singapore, #HongKong, & #NewZealand followed a similar path.
11/
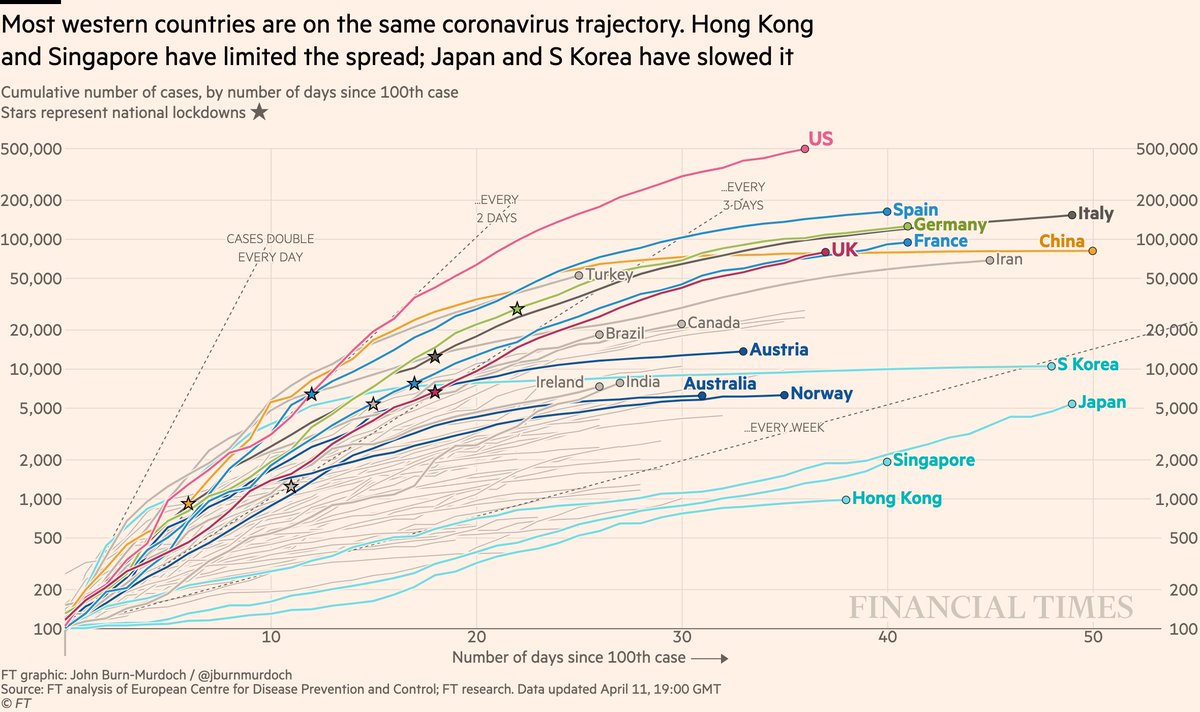
#Japan, #Australia, #Norway, #Finland, #Austria might be able to follow a similar path.
12/
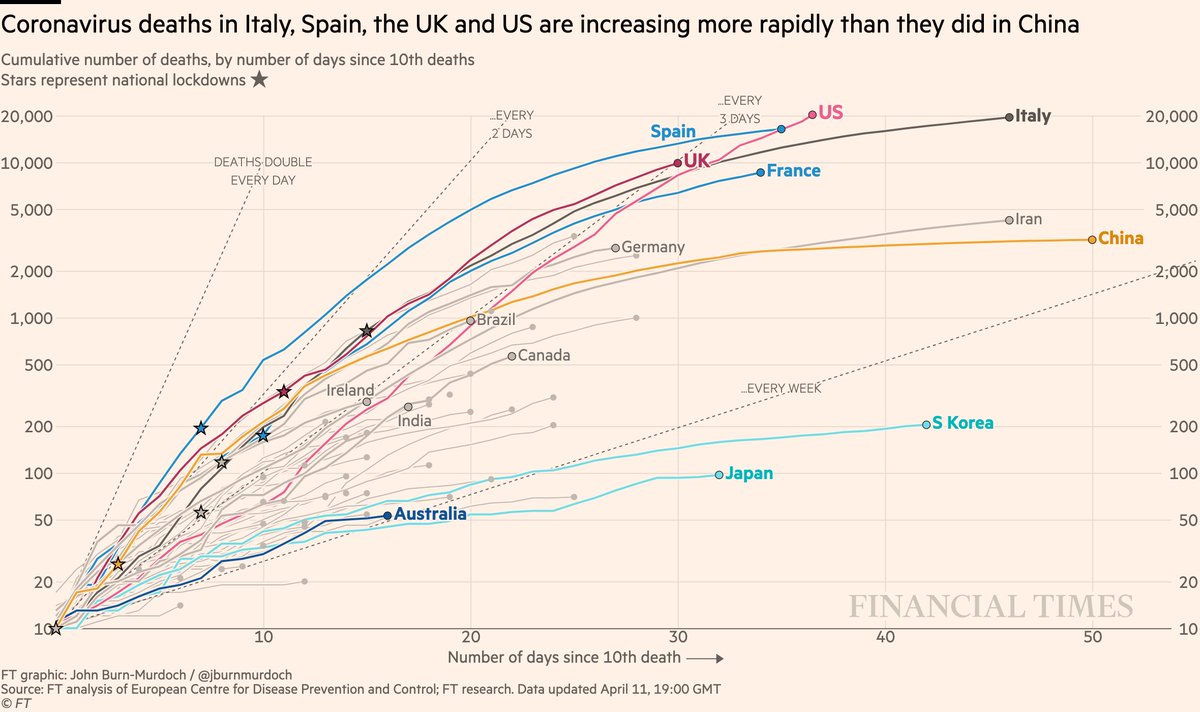
14/
15/
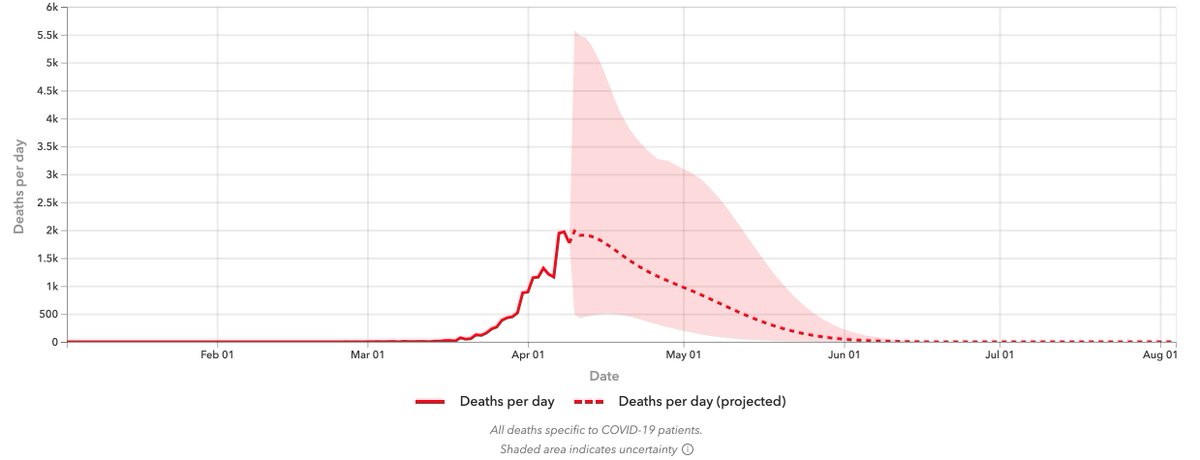
The #coronavirus has up to a 14 day incubation period. Then ~ two weeks of general symptoms (fever, fatigue, cough, etc).
16/
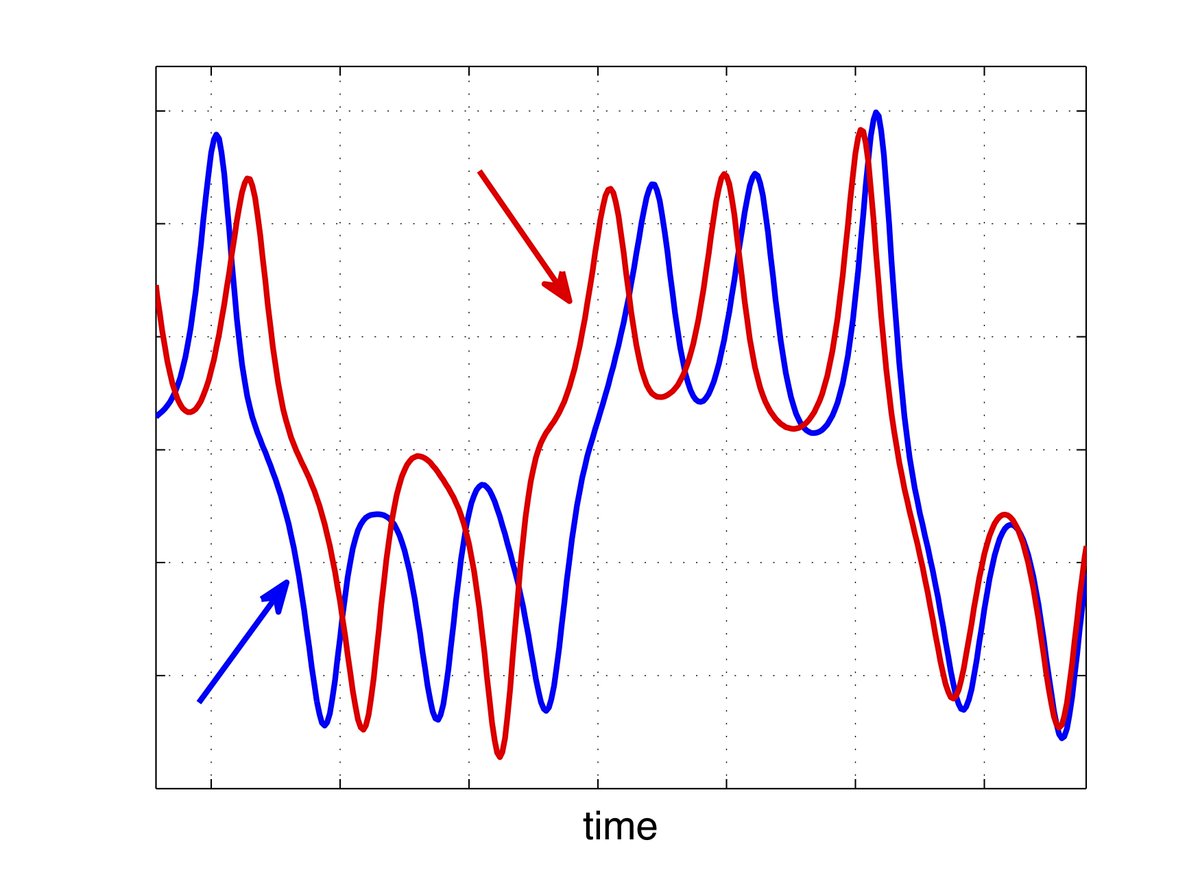
18/
19/
20/
21/
I did not build or use a sophisticated dynamic model for #COVID. We knew that interventions started late in the US. And that there is a ~4 week delay between the onset of lockdowns (March in US) and slowdown of exponential growth.
22/
+ : increasing
~0 : plateau
– : decreasing
26/
#Kinematics | #Epidemics
Acceleration | Slope of Daily Deaths
Velocity | Daily Deaths
Distance | Cumulative Deaths
27/
#Italy & #Spain grew to large daily numbers but now have – slopes.
#US & #UK: still increasing, +.
28/
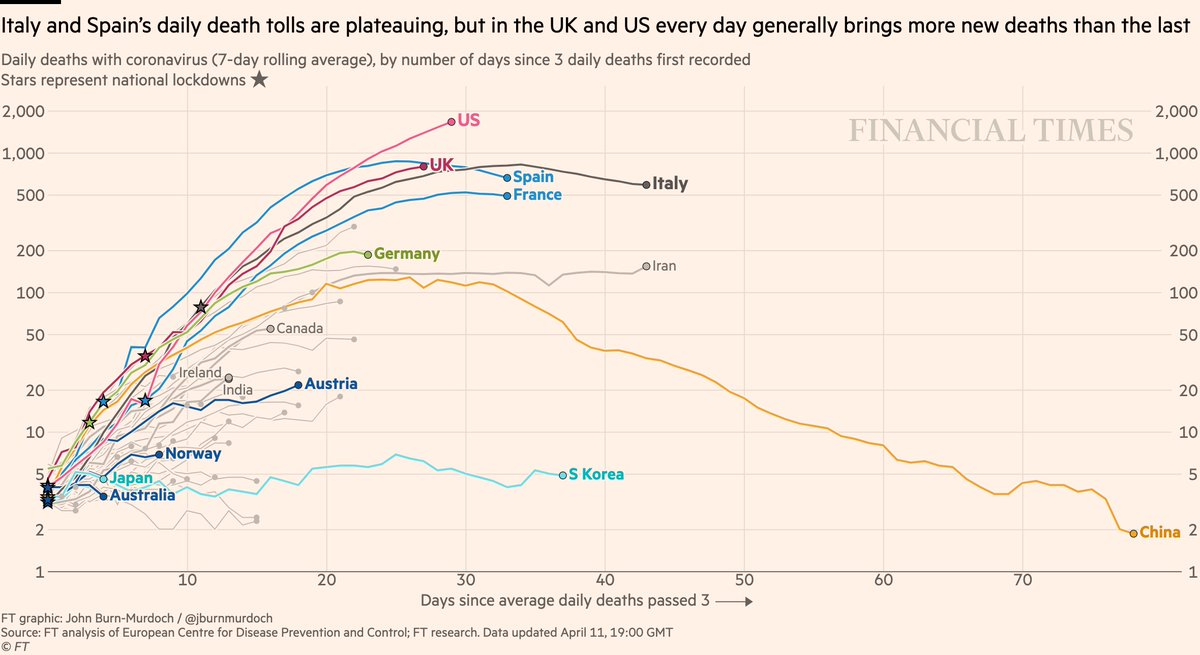
Below daily cases plot w Singapore & Hong Kong:
29/
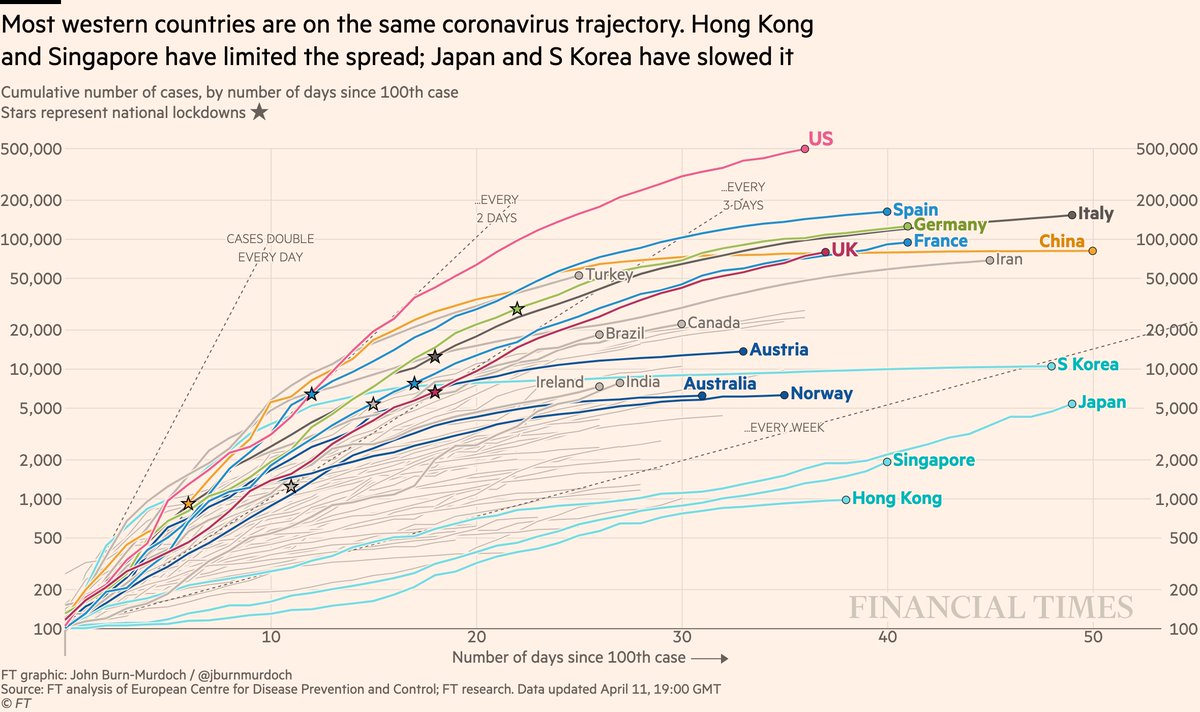
31/
#COVID deaths provide a more robust indicator of disease spread than cases, because identifying cases depends on the extent of testing, as it happened in many countries, including the US.
We had many cases in the US, but they were undetected.
32/
See, e.g., NYC and Bergamo:
33/
A big thank you to @FT #COVID Team and @jburnmurdoch for creating & updating their excellent plots, which I frequently use in my tweets.
34/
CC: @AydenLincoln