Thread! 🚨 (1/13)
atherosclerosis-journal.com/article/S0021-…
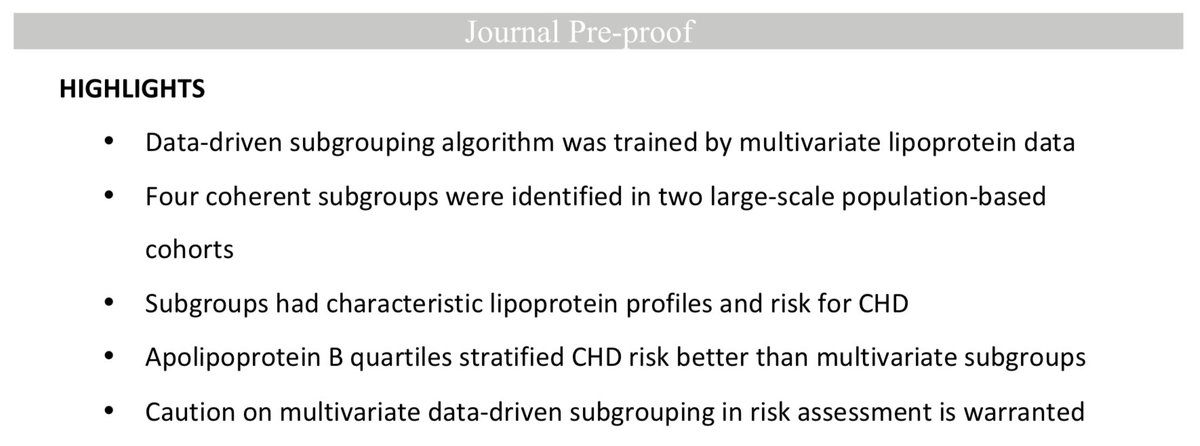
See: academic.oup.com/ije/article-ab… (2/13)
Keep Current with Pauli Ohukainen
This Thread may be Removed Anytime!
Twitter may remove this content at anytime, convert it as a PDF, save and print for later use!
1) Follow Thread Reader App on Twitter so you can easily mention us!
2) Go to a Twitter thread (series of Tweets by the same owner) and mention us with a keyword "unroll"
@threadreaderapp unroll
You can practice here first or read more on our help page!